What is a Data LakeHouse?
TL;DR: DataLakeHouse is an open source platform stack for Big Data Management that provides business value from Data Lake to Analytics. In order to reduce friction and provide thought leadership for organizations of all size, DataLakeHouse provides a pre-built stack architected from Big Data best practices and first-principles. These first-principles of a DataLakeHouse, provide data workers in an organization with the most promising step into modern Big Data Management and achieving Business Value (ROI) through their data. DataLakeHouse is a framework, platform, and a solution stack. Fundamentally, deploying a Data Lakehouse solution requires both technology infrastructure and architecture considerations.
It creates a data-value-chain starting at Data Lake ingress on one end of the architecture to analytical reporting outputs on the other end. Connecting the two sides of the architecture the DataLakeHouse stack includes Data Wrangling and pre-built Data Warehouse solutions. DataLakeHouse will be open to deploy on all major cloud vendor platforms as a means to proliferate its architecture as ubiquitously as possible. In effect, the data-value-chain concepts of the architecture are immediately understandable and achievable by any organization. And, the business value of the DataLakeHouse stack is visible through an analytics capability that is curated through subject matter experts across industries to accelerate Big Data Management integration for every organization, no matter its size.
Why have a Data LakeHouse?
Above all, the DataLakeHouse represents a concerted focus on achieving business value from your data. The definition of ‘value’ can vary from organization to organization, based on the nature and goals of each individual business’ operation. Like most organizations there is tremendous amounts of unlocked potential in their data. DataLakeHouse.io is a platform that provides immediate guidance on how to begin unlocking that potential.
Having a DataLakeHouse allows for first-principles in separation of duties across the data pipeline stack, i.e.: from ingest to egress to achieve that business value. From infrastructure, to governance, from self-service to production reporting, from data collection and model training to Machine Learning Production. That flow of data has many inlets, outlets, and workflows. Some of these are for obvious reasons more pronounced than some of the others. For example, a global sales team may heavily rely on their global CRM implementation, but not be so concerned about Human Resources (HR) data. But perhaps the global CRM implementation relies on some pieces of data from the HR data, for example compensation plan, % of sale incentive, etc. Then that data will need to be capture, stored, organized, protected, and shared for the purpose of helping achieve the global CRM implementation initiative’s goals.
The idea of KPIs (Key Performance Indicators) still heavily exists inside of a Data LakeHouse architecture. As do newer, common problems that the architecture solves for:
- Data Engineers and Data scientist developing in notebooks working on their local machines, but no idea where their work is, perhaps it’s not checked in to any source control, and the business side of the organization cannot leverage or gain visibility to the hours or days of work that has been done
- Business Value realization
- Path to Production
- Package, certify, roll-out in a controlled fashion, and determine business value, ROI to cost (is it worth the compute and man hours),
- Process Maturity Model (post deployment)
- Complete the circle, automated retraining, reproduce models and how to know when to retrain a model and continue the lifecycle
- Trust high quality data coming into the system
- Reproducibility of the work
- Automation
- MLOps
- CI/CD
- Analytics
What Qualities are part of a Data LakeHouse Architecture
DataLakeHouse believes there are 6 key principles behind providing an end-to-end Big Data Management solution for all organizations as a baseline data-value-chain:
- capture
- storage
- organization
- protection
- sharing
- value-producing
By meeting all of the key principles a DataLakeHouse concept is established. Most organizations have part of the solution. This is typical either because the organization is a late-adopter coming from legacy technology or that they have started the Big Data journey for a specific use case. DataLakeHouse seeks to fill the gap between these common a cases.
What does a Data LakeHouse Architecture Look Like?
The architecture is comprised of full, end-to-end platform structure specific to gaining business value from an organization’s data without the headaches of building a system from scratch or fumbling through the idea of necessary components required to align with best practices – it is the only Data Lake to Analytics solution most organizations will ever need:
- Infrastructure
- Secure Network
- Object Storage (aka Data Lake)
- Machine Learning Process Flow
- Source Control
- Data Science Collaboration
- Data Wrangling
- Data Virtualization
- Data Pipeline
- Data Warehouse
- Data Processing
- Data Analytics/Reporting
What can a Data LakeHouse do that a Data Lake Cannot?
Since a Data Lake does nothing by itself except store data in a distributed object storage system network, the DataLakeHouse as a principle of end-to-end data to analytics, is that it empowers your Data Lake Storage. By taking out the guess work of what a Data Lake is supposed to do for the business value of the Data Lake investment, DataLakeHouse brings life to data, in all aspects, without neglecting an organization’s choice of which cloud vendor should be selected to enable the architecture – it works on all major cloud vendors, and on-premise, so a company can have a Data LakeHouse platform to bring business value from any type of data.
What can a DLH do that a Data Warehouse Cannot?
A Data Warehouse is one aspect of a DataLakeHouse. Just like sales is a part of marketing, the belief is that decades of Data Warehouse development and refinement have shown us that real business value is achieved by answering the questions of decision makers by providing reporting and data based on their requirements. This curated dataset that is purpose build expedites results, creates speed to reporting, and saves time and effort otherwise spent compiling data. The ROI can be calculated for a Data Warehouse. And since the DataLakeHouse architecture provides pre-built reporting and Data Warehouse, and Data Lake artifacts and Data Pipelines, the business value is immediately apparent as well. The age old build vs. buy comes into play with DataLakeHouse. For companies that already have a Data Warehouse, fantastic, embrace the principles of Data Wrangling, Data Virtualization, Data Pipeline, etc. that’s stitches the architecture together. Already have a Data Lake? Great, then behold the other areas of the DataLakeHouse Architecture and best practices most data team’s are probably missing.
How Long Does it Take to Build a Data LakeHouse?
The key benefit is that you can build a project around your DataLakeHouse, just as you’ve done a previous project for DevOps, SRE, Data Warehousing, Custom Java Applications, or perhaps your latest attempt at building a “Data Lake”. This is technology, and DataLakeHouse is platform framework that provides an architecture for components to work together in a focused goal of providing business value quickly. The need for customizations and the size of each organization’s data, and data engineering team can vary which will impact time to value. All we can say is that it will definitely take less time than if you attempt to build each component of the architecture individually without the Data LakeHouse framework.
Made with Glorious Purpose in the Carolinas
We ❤ Data Teams.
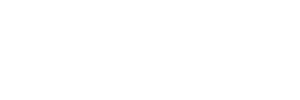
DLH.io (aka DataLakeHouse.io) is an Enterprise Data Integration and Data Orchestration platform built to deliver your data consistently and reliably. This data synchronization process provides the data pipelines, for target integrations in your Data Lake, Data Warehouse, or Data Lakehouse. It’s ELT and ELT for the modern data stack and much more.